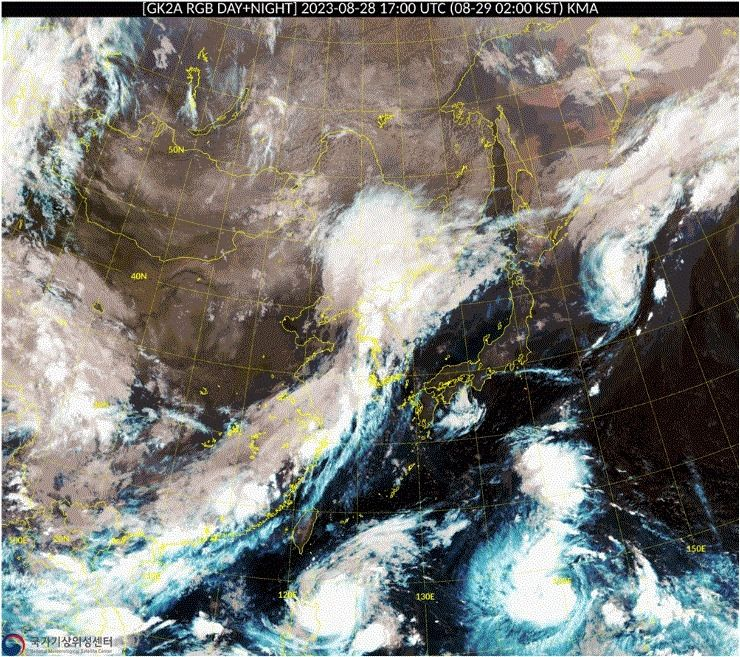
A team led by Professor Im Jung Ho of the Department of Earth and Environmental Urban Engineering at the Ulsan National Institute of Science and Technology (UNIST) has developed a technology predicting the intensity of typhoons with advanced precision combining real-time satellite data and deep learning technology. The Hybrid Convolutional Neural Network (Hybrid-CNN) model objectively extracted environmental factors affecting changes in typhoon potency and predicted the typhoon intensity up to 72 hours later.
Hybrid-CNN technology can also be applied to the current forecast system, providing forecasters with quick and accurate typhoon information, which will be of great help in disaster preparedness and damage prevention.
According to UNIST on the 2nd, the typhoon intensity prediction model combined geostationary weather satellite data and numerical model data to show improved performance by about 50% compared to existing forecasts. It boasted excellent forecasting performance even in situations of the typhoon rapidly strengthening.
The Hybrid-CNN model developed by the research team objectively and accurately predicts the typhoon intensity after 24 hours, 48 hours, and 72 hours.
The general typhoon observation method was mainly analyzed by forecasters using only geostationary satellite data. It took a long time to analyze the data with the disadvantage of depending on the uncertainty of the numerical model. However, the model developed by the research team can provide more accurate typhoon forecasts by reducing the uncertainty of the numerical model thanks to greatly increased analysis speed.
The research team used transfer learning with Chollian 1 and Chollian 2A satellite data to estimate the typhoon intensity. The experts employed AI to automatically visualize and quantitatively analyze the typhoon intensity, aiming to enhance the accuracy of typhoon forecasts. The AI that learned the existing weather data quickly and accurately analyzed the new typhoon data.
Im said, “The deep learning-based typhoon prediction framework will enable forecasters to provide more accurate prediction information, allowing for quick and effective measures.”
Most Commented